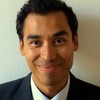
Gilberto Batres-Estrada
Webstep AB, Sweden
Title: Long short term memory networks: Theory and applications
Biography
Biography: Gilberto Batres-Estrada
Abstract
A Recurrent Neural Network (RNN) is a type of neural network suited for sequential data. Its recurrent connections from one time step to the next introduces a depth in time, which in theory, is capable of learning long term dependencies. There are however two serious issues with vanilla RNNs, the first is the problem of exploding gradients, where the gradient grows without bound leading to instabilities in the system. Growing gradients can in part be alleviated by a technique known as clipping gradients. The second problem is that of diminishing gradients, which has no solution for the vanilla RNN, but for some special cases. The Long Short Term Memory Network (LSTM) is a type of RNN designed to solve both of these issues, experienced by RNNs during the training phase. The solution lies in replacing the regular neural units in an RNN with units called memory cells. An LSTM memory cell is composed of four gates controlling the flow of the gradients which dynamically respond to the input by changing the internal state according to the long term interactions in the sequence. The LSTM has been shown to be very successful at solving problems in speech recognition, unconstrained handwritten recognition, machine translation, image captioning, parsing and lately in prediction of stock prices and time series prediction. To combat over-fitting, techniques such as dropout have become standard when training these models. We start by presenting the RNN’s architecture and continue with the LSTM and its mathematical formulation. This study also focuses on the technical aspects of training, regularization and performance.