Day 2 :
Keynote Forum
Boris Stilman
University of Colorado & STILMAN Advanced Strategies, USA
Keynote: Introduction to the primary language: Discovering the algorithm of discovery
Time : 09:30-10:10
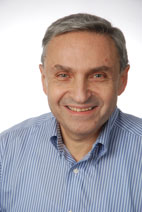
Biography:
Abstract:
Keynote Forum
Steve Wells
Fast Future Publishing Ltd, UK
Keynote: Going beyond genuine stupidity to ensure ai serves humanity
Time : 10:10-10:50
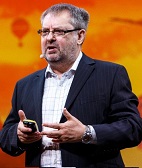
Biography:
Abstract:
Keynote Forum
Mihaela Ulieru
IMPACT Institute for the Digital Economy
Keynote: Personal analytic engines through blockchain: disrupting big data
Time : 11:00-11:40
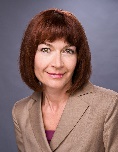
Biography:
Abstract:
- Artificial Intelligence | Artificial Neural Networks | Deep Learning | Bioinformatics | Computational Creativity | Parallel Processing | Self Organising Neural Networks
Location: Laine
Session Introduction
Dean Armitage
The Farsighted Company, USA
Title: Is the dawn of singularity the sunset for humanity: The need for singularity oversight for the continuance of humanity
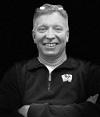
Biography:
Abstract:
Esteve Almirall
Ramon Llull University, Spain
Title: From competing on analytics to competing on models
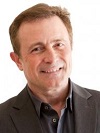
Biography:
Abstract:
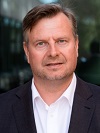
Biography:
Abstract:
Andrea Burgio
ESIEE School of Engineering, France
Title: Markov meets Schrödinger: rebranding uncertainty and the fear factor
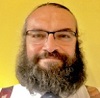
Biography:
Abstract:
- Cognitive Computing | Natural Language Processing | Percetrons | Backpropagation
Location: Laine
Session Introduction
Erwin E Sniedzins
Mount Knowledge Inc., Canada
Title: Using microself-reinforcement learning, Nlp and AI gamification in dynamic real-time
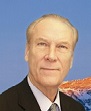
Biography:
Abstract:
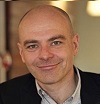
Biography:
Abstract:
Introducing AI and ML technologies into the enterprise, as with any IT project, has a number of challenges. A number of these are often comparable to the delivery of a standard IT project, such as ensuring there is a clear business outcome, a defined scope for delivery, stakeholder engagement, a suitably qualified team and the ability to measure progress as the project continues. However, there are subtle differences that if not addressed or the business and technology stakeholders are not made aware, can increase the risk, cause issues during delivery and ultimately and potentially cause the project to fail. With a muchhyped technology that is immature in its wide-scale enterprise adoption such as AI, a high-profile failure can set an organization back in its appetite to adopt this technology again in the near to medium term. The aim is to highlight some of the issues that are likely to arise, from understanding the organizations strategy for AI, structuring a project delivery approach, engagement with users, ensuring that you have the right mix of resources to deliver, how to integrate with wider systems, security, privacy and data controller concerns and subsequently some proven approaches to take in addressing these.
Gilberto Batres-Estrada
Webstep AB, Sweden
Title: Long short term memory networks: Theory and applications
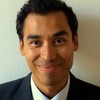
Biography:
Abstract:
Jivitesh Sharma
University of Agder, Norway
Title: Deep Neural Network system for Fire Emergency: Detection, Analysis and Evacuation
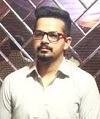
Biography:
Abstract:
Patricio Julian Gerpe
Argentine AI Community, United Kingdom
Title: An Application of Agent-oriented Programming to connect Rural Merchants in Ethiopia with on-demand transportation
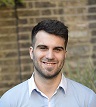
Biography:
Abstract:
Biography:
John Kontos has a PhD from Birmingham University in Computers. He has published over 100 papers, among other topics, in Natural Language Processing, Deductive Question Answering, Explanation Systems and Machine Learning. He has headed the design and construction of the first Greek digital general purpose computer. He has served as Full Professor of AI at the Economic University of Athens and at the University of Athens.
Abstract:
The poster is proposed to conceptualize different Machine Learning Methods, e.g. the ANN-based ones, as equivalent to different String Matching methods for retrieving from a table of examples. The aim of the proposal is to clarify what Machine Learning methods can be reduced to in order to avoid the anthropomorphic concept of Machine Learning. Such a reduction may also help in converting “learned” systems to “explainable” systems that enhance trust. The table below shows a correspondence between String Matching methods and Machine Learning methods.
String Matching method Machine Learning method
1. Sequential Serial
2. Non-sequential Serial (Decision Trees (DT))
3. Weighted Total Parallel (Single ANN)
4. Weighted Partial Parallel (Multiple ANNs)
5. Hierarchical Weighted Partial Parallel (“Deep” ANNs)
First we notice that Method No.2 differs from No.1 in the different order of symbol by symbol matching procedure. This change of order by computing a DT (e.g. ID3) is meant to reduce the computing effort necessary for matching between a set of examples and a string under test. Since methods Nos 3 to 5 according to recent literature may be reduced to DTs we may consider them as variants of serial string matching. Future work must analyze the “unseen” example cases.